|
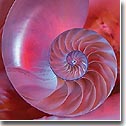 |
|
|
|
|
Course |
CEE 700/800 CEE Experimental Methods
Lecture 3 hours; 3 credits
Spring Semester, 2025
(offered Face-to-Face & Online)
Course Website: http://asellus.cee.odu.edu/expdesign/
-
CALL No.: 21293
CEE 700 - ODU Main Campus (ODU), Gornto 205
CALL No.: 31053
CEE 700 - Hamptoon Roads area (WC2)
CALL No.: 31055
CEE 700 - In Virginia (WC5)
CALL No.: 31056
CEE 700 - Outside of Virginia (WC7)
CALL No.: 21294
CEE 800 - ODU Main Campus (ODU), Gornto 205
CALL No.: 31058
CEE 800 - Hamptoon Roads area (WC2)
CALL No.: 31061
CEE 800 - In Virginia (WC5)
CALL No.: 31062
CEE 800 - Outside of Virginia (WC7)
|
Session |
Spring; January 11 (Sa) through April 28 (M), 2025
|
Time/Days |
Tuesday, 7:25 PM-10:05 PM |
Classroom |
Gornto Distance Learning Building, Gornto 205
and Online
|
Prerequisite |
MATH 212 (Calculus II)
Students are presumed to have a good foundation in mathematics
and reasonable understanding of programming logic.
Mildly DISCOURAGED
if a student has finished *less* than
a minimum of 6 credits of graduate coursework.
|
Instructor |
Jaewan Yoon, Associate Professor, CEE,
KH 130 |
Phone |
(757) 683-4724 |
Fax |
(757) 683-5354 |
e-mail |
<jyoon@odu.edu>
|
|
|
-
"Clarity in logic, augmented by deductive processes."
"To make a correct and objective inference about the
System (=Population) based on information contained in
Experiments (=Samples)."'
"With its Reproducibility confirmed and verified, engineering
design then signifies entelechial Scalability."
The objective of this course is to provide graduate-level students (1)
an overview of various experimental designs and problem
conceptualization methods used in research and in the real-world
environment
(i.e., "See the Forest not just Trees" -- it's all about the SYSTEM!!),
and (2) proper and practical statistical methods applicable
to multidisciplinary engineering problems with an environmental
engineering stress.
Fundamental and general concepts on system characterization adn statistics
will be reviewed in the beginning part
of the semester, then progresses rapidly into various statistical methods applicable to research/project
activities such as experiment/sample design strategies, test of hypotheses, and the
analysis aspect including regression and correlation, analysis of variance, nonparametric
statistics, and probability distributions -- to consummate "Why would/should I" prior- and
"What am I going to do with" posterior-conditions.
Emphasis will be equally on concepts and applications. Statistical
Analysis Systems (SAS) software available in
ODU MoVE (Monarch Virtual Environment/VM)
(for interactive process) and
ODU HPC (High Performance Computing) cluster
(for batch process) platforms will be heavily
used for assignments and for the class project.
|
|
-
Textbook
|
Applied Statistics and Probability for Engineers,
7th Ed.,
(2018), Douglas C. Montgomery and George C. Runger, John Wiley & Sons,
ISBN: 978-1-119-57061-5 (Hardcover);
ISBN: 978-1-119-40036-3 (eBook)
|
|
|
-
- Descriptive Statistics - Measure of Location (C.T.),
Measure of Variability (Disp.), Measure of Symmetry (Skewness)
and Measure of Peakedness (Kurtosis) -- reconstructing
images of the population via sample(s).
- Probability - Interpretation of Probability, Probability Rules,
Bayes Theorem applications.
- Discrete Random Variables and Probability Distributions -
PDF, CDF, Binomial & Poisson Distribution.
- Continuous Random Variables and Probability Distributions -
Normal Distribution and other Normality-based PDFs.
- Joint Probability Distributions - Discrete vs.
Continuous Random variables, Chebychev's Inequality,
Shapiro-Wilk and Kolmogrov-Smirnov tests.
- Point Estimation - Statistical Inference, Maximum
Likelihood Method, Chi-square, t-, and F-distributions.
- Interval Estimation - Confidence Interval, Tolerance Interval
- cover all but stretched thin, or cover less but thick and sturdy,
that is the question.
- Test of Hypothesis (T.H.) - What you see is What you get, really?
Let's double-check by using T.H. - p-value, Type,
Case, Do and Don't of Hypothesis Testing.
- Nonparametric Statistics - Sign Test, Wilcoxon Rank Sum
Test, Kruskal-Wallis Chi-Square similitude, Asymptotic sample approximation,
Friedman's Nonparametric ANOVA.
- Linear Regression and Correlation - Least Squares
Estimation, Significance of Regression, Coeff. of Determination,
Mean Squared Error (MSE) and RMSE analysis.
- Multiple Linear Regression - Least Squares minimization technique,
Residual SS and variance analysis, Multivariate minimization,
R2 vs. Adjusted R2,
Stepwise regression and Mallow's Cp statistics,
Multicollinearity.
- Principal Component Analysis (PCA) - Dimensionality-reduction method
for large data sets with variance-covariance linear combinations.
Pre-screening and idetification of key reproducibility variables
that are strongly correlated.
- Single-Factor Experiment Design - Analysis of Variance (ANOVA),
Residual Analysis, CR and RCB Designs, Tukey's LSD and
Duncan's MRT Multiple Means Comparison (MMC) methods.
- Multiple Factorial Design - Orthogonal Latin Square
and Factorial Experiments,
Replication, Randomization and Blocking, Response Surface Methods,
Factorial Multicollinearity. GLMSelect technique and subsequent
Heteroscedasticity of predictive model(s).
Also
- Normality, normality, and normality. Assumption vs. Verification.
- How the test of hypothesis (T.H.) works - correct hypothesis vs. induced hypothesis.
- Good, Bad and Ugly T.H. -- a decomposed, "singular" hypothesis with idiotic simplicity
vs. a tangled, "complex" hypothesis with unnecessary multiplicity.
(Eschew obfuscation!)
- The magic silver bullet - dealing with p-value (are you sure
about your conclusion?)
- Comparison of Means - Are they different and how sure are you? How
different are they? And the implication -- see the forest not trees.
- Graphical Data Analysis - Why do we use graphs in the first place?
Often-used bad graphs vs. rarely-used good graphs.
- How to build a correct & good (or so-so) regression model,
and when you should not even attempt to build one.
Common misunderstanding, gross abuse and true meaning and
applicability of regression techniques.
- Handling data below detection limits (now you see, now you don't).
- Dealing with outliers (Gremlins!) - to include or not to include,
that is the question.
- When do you should consider data transformations?
Would it biased?
- Trend analysis - Are things getting better or worse?
Gradual trends vs. quick changes.
Am I seeing a temporal cyclic recurrence?
-- Spectral analysis, Time series and Autoregressive models.
- All mighty Nonparametric statistics!!!
- Using control charts and confidence intervals - What's
the difference? When to use which?
- Control Charts - Boundary conditions of QC/QA -- the cost
of "quality" and its propagated ramification and liability.
|
|
|
|
-
-
"Memorization is a function
of duty; Knowledge comes from the inquisitive mind."
- Throughout this course, I will encourage you to think, to reason,
and to question. I will stress knowledge of and application
of broad general concepts because these are all you will
remember 2, 5, or 10 years from now. However, one still needs
to know the details of these concepts, but generally you can
look up the details after you recognize the concept.
- The classroom presentation will be a combination of lecture
and discussion. I
will frequently ask questions during lectures to promote
discussion of various topics and to stimulate thoughts.
Feel free to interrupt me at any time in order to discuss
points that you do not understand or simply want to discuss in more
detail. (of course, at the same time we need to use
the time wisely to cover class topics)
Remember, I will not be able to guess which topics they
might be -- you must tell me first. The only dumb questions
are those that are never asked and left in the dark.
- Somewhat a disturbing concept yet the more you make mistakes (or
downright embaress yourself upon incorrectly answering
questions asked by the instructor), the more you will learn (and remember,
not the embaressment part I hope).
I.e., class participation is critical for your true learning process.
The very nature of topical coverage requires ascertaining
your understanding of the topic as well as ascertaining that
you can equally state your understanding of the topic.
Don't be afraid, it is still a controlled flight afterall,
and there will be no casualty left behind by making incorrect
answer(s) in class -- please do consider taking an advantage of
such circumstance.
- Office Hours:
You're welcome to e-mail your question to me at
<jyoon@odu.edu> anytime.
Please e-mail
some explicit questions that demonstrate that you have
done some thinking about your problem on your own. You must
have a clarity on what it is that you
don't understand!
- Class website will be used to provide
your latest cumulative grade information.
Please make a habit to check the site often.
- Reasonable accommodations will be provided for students with disabilities.
Students should self-identify to me as early in the semester as possible.
|
|
-
Project |
50% |
Final Project Report dues by May 2 (Friday),
5:00 PM or earlier via Canvas/Assignments |
Midterm Exam |
20% |
Takehome exam,
exam material will be posted to Canvas/Assignments
on March 5, Tuesday 7:00 PM. Due by
March 7 (Thursday) 5:00 PM or earlier via
uploading your solution to Canvas/Assignments
|
Final Exam (Comprehensive Oral Exam) |
30% |
April 29 (T) and April 30 (W).
20-min. per student via Zoom.
==> Sign-up and schedule your comprehensive oral final exam in advance.
|
|
|
|
Total |
100% |
|
- Though there will be no explicit homework assignment,
problems will be assigned and solution will be posted to Canvas in one-week cycle.
- For PhD students who had registered for CEE 800, in addition to
"all above," you are required to submit a term research papers
(due together with your Final Project Report). Topic
of the term research paper will be identified after a short meeting
with the instructor in the beginning of the semester.
 |
Final Grade Assignment
|
-
Letter grades will be based on brackets (see right side) out
of the normalized 100% total.
A cumulative total equals
to a 65 percentile or higher will guarantee you a grade of C- or
better. A cumulative total smaller than a 65 percentile will
guarantee you a grade of F. No grading based on the curve.
|
|
100%-93% |
A |
< 93%-88% |
A- |
< 88%-85% |
B+ |
< 85%-81% |
B |
< 81%-78% |
B- |
< 78%-73% |
C+ |
< 73%-68% |
C |
< 68%-65% |
C- |
< 65% |
F |
|
 |
Grading Criteria
|
-
Key is that you should solve problems clean and tight with
your assumption(s) clearly stated in the beginning.
For example, if a typical problem worths a total of 10 points,
it would be graded using following criteria;
|
-
Technical solution (8 points total) |
|
|
What it meant to be (Completely correct)
|
|
8/8 |
Approach is o.k., but a wrong answer/conclusion, a.k.a., a computational error
or/and an unit conversion meltdown situation
|
|
6/8 |
Reasonable attempt (yup, it shows), but plagued by serious error(s)
|
|
4/8 |
Poor effort, perfunctory and incomplete,
however still trying to show that you are trying
|
|
2/8 |
Problem not attempted
|
|
0/8 |
Given answer(s) alone is correct by itself yet completely irrelevant
and superfluous so that the answer has nothing to do with the
solution asked/required by the question
|
|
-2/8 |
Does not appear to be written/stated by an engineering major
|
|
-4/8 |
Does not appear to be written/stated by a graduate student
|
|
-20/8 |
|
Presentation (2 point total) |
|
|
Neatly and succinctly illustrates one's thought process and
rationale behind procedures (which can be correct or incorrect)
|
|
2/2 |
Murky as well as in Dead Sea Scrolls quality,
yet still theoritically traceable/decipherable
with darn good efforts based on profiling of the individual's idiosynchracy
|
|
1/2 |
Starts causing throbbings in the sinus
|
|
0/2 |
Causes a severe migraine headache, illegible
|
|
-2/2 |
Does not appear to be written/stated by a graduate student
|
|
-10/2 |
|
 |
Logistics
|
-
- Though there will be no explicit homework assignment,
problems will be assigned and solution will be
posted to Canvas in one-week cycle.
-
Takehome Midterm Exam material will be posted to Canvas/Assignments on
March 11, Tuesday 7:00 PM.
Due by March 13 (Thursday) 5:00 PM or earlier via your PDF solution upload to
Canvas/Assignments.
- Comprehensive Oral Final exam will cover
*all* the topics discussed during the semester in a format
that the examinee will examine and pick one
out of multiple scenariorized questions, then
logically identify where to start, what to, and how to analyze in order to derive
which necessary information for the problem, and
how to present the conclusion in a
objective and correct manner -- "real-world" approach,
do it correctly and use it correctly.
Final exam will be given on April 29 (T) and April 30 (W), and
it will be a 20-min comprehensive oral test per student
via Zoom.
==> Sign-up and schedule your comprehensive oral final exam in advance.
- If you think your exam was incorrectly graded for some
arguable reason, please return it to me with a detailed explanation.
I reserve the right to completely regrade an exam that
has been returned. (Regraded result can be either better or worse
than the original grade).
- For statistical analysis on larger daaset, SAS (Statistical Analysis System)
application in ODU MoVE VM server will be used.
SAS is de facto the statistical
software used by pretty much all professional and official domains.
Most importantly, if you can interprete SAS output/Listing, you can
interprete outputs from any variety of statistical software
with confidence and clarity since
they all rooted from SAS and use the same/similar output format and
syntax. Thus by learning SAS, you become capable of using any other
statistical software, correctly and effectively.
This does not necessariy prevent you from using other statistical software
(such as R, SPSS, JMP, Minitab, MatLab, etc.) for assignment and
and your project research. Key is that you
should be able to interprete the analysis results (from whichever
statistical software that you used), not just be able to run analysis
(without receiving any error).
- For any SAS assignment, always add/put
your interpretation or conclusion on SAS analysis
results rather than just bring SAS
output listings. (Think "why
did I run SAS for in the first place?")
Always keep in mind that just pushing a bunch of numbers
under someone's nose without clearly interpreting the
implication(s) would be an abominable vice as an engineer.
Do eschew obfuscation!
|
 |
Class Project
|
-
"I see!", said the blind carpenter, as he picked up his hammer and saw.
-- William Shakespeare, MacBeth
- One of requirements for this course is that each student conducts a
small statistical design/analysis project related to a research topic(s)
in one's field of study, i.e., environmental, structural,
geotechnical, water resources, coastal, transportation, biofuel,
modeling, sampling, lab experiment, etc.
- Idea is that by conducting a small-scale statistical/experimental design
class project on your research topic(s), you can readily apply similar
methodology/technique(s) to or later develop the very idea/concept
into your own dissertation/thesis/project research -- i.e., do a
"seed idea project.".
This would be
tremendously beneficial for your current/future research,
especially in the areas of hypothesis testing,
comparison of data (either mean or variance or both), regression analysis,
sampling strategy, etc. -- so that the experimental design techniques will
*help* you toward accomplishing your research goal, *not* becoming
your research goal.
- I can guarantee you that you'll get all the necessary helps and plus
from me throughout the entirety of your project. If you feel stuck somehow,
do not procrastinate, go ahead and request for an advisory
meeting in regard to your project idea/problem!
- Each student is required
to submit a full Final Project Report (no hardcopy report,
single PDF file submitted/uploaded to Canvas/Assignments)
for one's project.
Guidelines for the
project/report element and format as well as its grading criteria
are also available in the class website.
Final report is due by May 2 (Friday) 5:00 PM
via uploading to Canvas/Assignments
- Be serious about the deadline!! -- (if it
is not that serious, people wouldn't put the word 'dead' in the first
place, isn't it?) Late project report submission will be accepted with
a 30% deduction, i.e., your report will be
graded based on a maximum point of 70.
- Remember, the ability to deliver a thorough, technically-sound, and
quality project on-time is what distinguishes you from the undergraduates and
what distinguishes the engineers from the scientists(!).
|
 |
Honor System
|
-
The Old Dominion University Honor Code applies to all works associated with this course.
Honor the Honor Code. Remember, the academia is all about
pride and
respect, and the
Honor Code is the heart of it. Think about it, really.
|
|
CEE 700/800 CEE Experimental Methods
Lecture 3 hours; 3 credits
Spring Semester, 2025 (January 11 - April 28, 2025)
CRN_CEE 700: 21293, 31053, 31055, 31056
CRN_CEE 800: 21294, 31058, 31061, 31062
Day/Time: Tuesday 7:25-10:05 PM @ GORNT 205 & Online
-
Today is
 |
Important Dates to Remember
|
Introduction to SAS
|
March 4 (Tuesday)
How to access SAS via ODU MoVE VM server,
and how to get your analysis done.
|
Midterm Exam |
March 11-13 (Tuesday-Thursday)
Takehome Test
Exam material will be posted to Canvas/Assignments on
March 11, Tuesday 7:00 PM.
Due by March 13 (Thursday) 5:00 PM or earlier via Canvas/Assignments.
|
1-page Project Proposal Due |
March 18 (Tuesaday) |
1-page Project Progress
Report Due (optional) |
April 8 (Tuesday) |
Final Exam |
April 29 - April 30 (Tuesday-Wednesday)
30-min. per student via Zoom
- April 29 (T) 7:00-9:00 PM
- April 30 (W) 7:00-9:00 PM
==> Sign-up and schedule your comprehensive oral final exam in advance.
|
Final Project Report Due
(and Term Paper for CEE 800) |
May 2 (Friday) by 5:00 PM or earlier,
via Canvas/Assignments
|
|
Class |
Date |
|
|
Topics |
Chapters |
1 |
1/14 |
T |
|
Introduction and General Overview,
the "Lemma of Reproducibility."
Frequently used and important
Descriptive statistics on CT and Disp --
Measure of Location (C.T.), Measure of Variability
(Disp.), Measure of Symmetry (Skewness) and Measure
of Peakedness (Kurtosis) in the "System."
|
1 and 6, Cookbook |
2 |
1/21 |
T |
|
(Joint|Conditional) Probability, and
applications of Bayesian Theorem with
Probability Tree Diagram technique.
|
2 and 5, Cookbook |
3 |
1/28 |
T |
|
Discrete Random Variables and Probability
Distributions - PDF, CDF, Binomial & Poisson,
and
Spatiotemporal Poisson Modeling techniques
and thier limitations.
|
3, Cookbook |
4 |
2/4 |
T |
|
Continuous Random Distributions -- Pervious
nature of Normal Dist. and CLT assumption (and
Chebychev's Inequality).
Normality validation with Shapiro-Wilk &
Kolmogorov-Smirnov Theorems.
Statistical Inference and Maximum Likelihood Method.
|
4, Cookbook |
5 |
2/11 |
T |
|
PDF/CDF & Interval Estimation -- Issue of
Density and Applicability. Concept of
inequality Constraints and Penalty functions used in
Confidence Interval applications.
|
4, 7 and 8, Cookbook |
6 |
2/18 |
T |
|
Test of Hypothesis -- Method of Deduction,
and Clarity in Logic
and consequent Decision-making processes
--
all based on p-value. TH is the Alpha
and Omega of all statistical methods.
What you see is What you get, really? Let's
double-check by using p-value, Do and Don't
of Hypothesis Testing.
|
4 and 9, Cookbook |
7 |
2/25 |
T |
|
All-mighty Nonparametric Statistics and its Applications,
Sign, Kruskal-Wallis and Wilcoxon's Rank Sum models
|
9 and 10, Cookbook |
8 |
3/4 |
T |
|
Introduction to SAS -- how to access SAS via ODU MoVE VM server,
and how to get your analysis done
|
SAS 101 |
9 |
3/11 |
T |
|
Midterm Exam
Open Book/notes.
Exam material will be posted to Canvas/Assignments on
March 11, Tuesday 7:00 PM.
Due by March 13 (Thursday) 5:00 PM or earlier via submitting/uploading
to Canvas/Assignments
(Spring Break, March 10(M)-15(Sa))
|
 |
10 |
3/18 |
T |
|
Univariate & Multivariate Regressions,
and Trigger/Response dependent linearity in a system.
Least Squares Estimation, Significance of Regression,
Coeff. of Determination, Mean Squared Error (MSE)
and RMSE analysis. Residual SS and variance analysis,
Multivariate minimization, R-sq vs. Adjusted R-sq,
Stepwise regression and Mallow's Cp statistics,
Multicollinearity.
1-page Project Proposal Due
|
11 and 12, Cookbook |
11 |
3/25 |
T |
|
General Linear Model (GLM), Higher-order
Nonlinear Polynomials and Stepwise Selection Scheme,
Various linearization techniques
-- what (really) it is
and what you could (incorrectly) do with it, and
the significance of p-value in all.
|
11 and 12, Cookbook |
12 |
4/1 |
T |
|
Analysis of Variance (ANOVA),
Single-Factor Experiment Design
-- what it is
and what you can (correctly) do with it, and yet again,
the significance of p-value in all.
Principal Component Analysis (PCA) -
Dimensionality-reduction method for
large data sets with variance-covariance
linear combinations. Pre-screening and
idetification of key reproducibility variables that
are strongly correlated.
|
13 and 14, Cookbook |
13 |
4/8 |
T |
|
Completely Randomized (CR) and
Randomized Complete Block (RCB) Designs
- RCB as a versatile Swiss Army knife, which actually can cut.
1-page Project Progress Report Due
|
13 and 14, Cookbook |
14 |
4/15 |
T |
|
Latin Square (LSQ) and Factorial Designs,
and Multicolinearity -- to find and evaluate
intrinsic 'clicks'. Further on Response Surface Methods,
Factorial Multicollinearity.
|
13, 14 and 15, Cookbook |
15 |
4/22 |
T |
|
Multiple Means Comparison
(MMC) techniques with Duncan's MRT and Turkey's LSD,
GLMSelect technique
and subsequent Heteroscedasticity of
predictive model(s).
|
13, 14 and 15, Cookbook |
|
|
|
|
|
|
* |
4/29-4/30 |
T-W |
|
Final Exam (Comprehensive Oral test)
30-min. per student via Zoom
- April 29 (T) 7:00-9:00 PM
- April 30 (W) 7:00-9:00 PM
==> Sign-up and schedule your comprehensive oral final exam in advance.
|
|
* |
5/2 |
F |
|
Final Project Report Due
(and Term Paper for CEE 800)
submitted/uploaded to Canvas/Assignments
by 5:00 PM or earlier
|
|
|
|
|
|